AI’s appetite for quant strategies
Last week US markets and crypto rose after the FED revealed they consider cutting interest rates in 2024. Market-implied probability based on CME Group 30-Day Fed Fund futures prices estimates that the FED will cut rates by March 2024 with a probability of more than 71%. Looser monetary policy was historically strongly correlated with rising Bitcoin prices.
A loosened monetary policy coupled with media campaigns around potential ETFs of various established institutions with large marketing budgets such as Blackrock, Fidelity, VanEck, Wisdomtree, Valkyrie, which will vie for future clients, in addition to the media attention around Bitcoin halvening could be the perfect storm for an enormous bull market. We are glad to say that our models have been capturing the positive trends effectively in the last quarter of this year and make us look ahead optimistically if markets continue to rise.
Nonetheless, despite the recent crypto runup Bitcoin volatility remains low compared to historical figures:
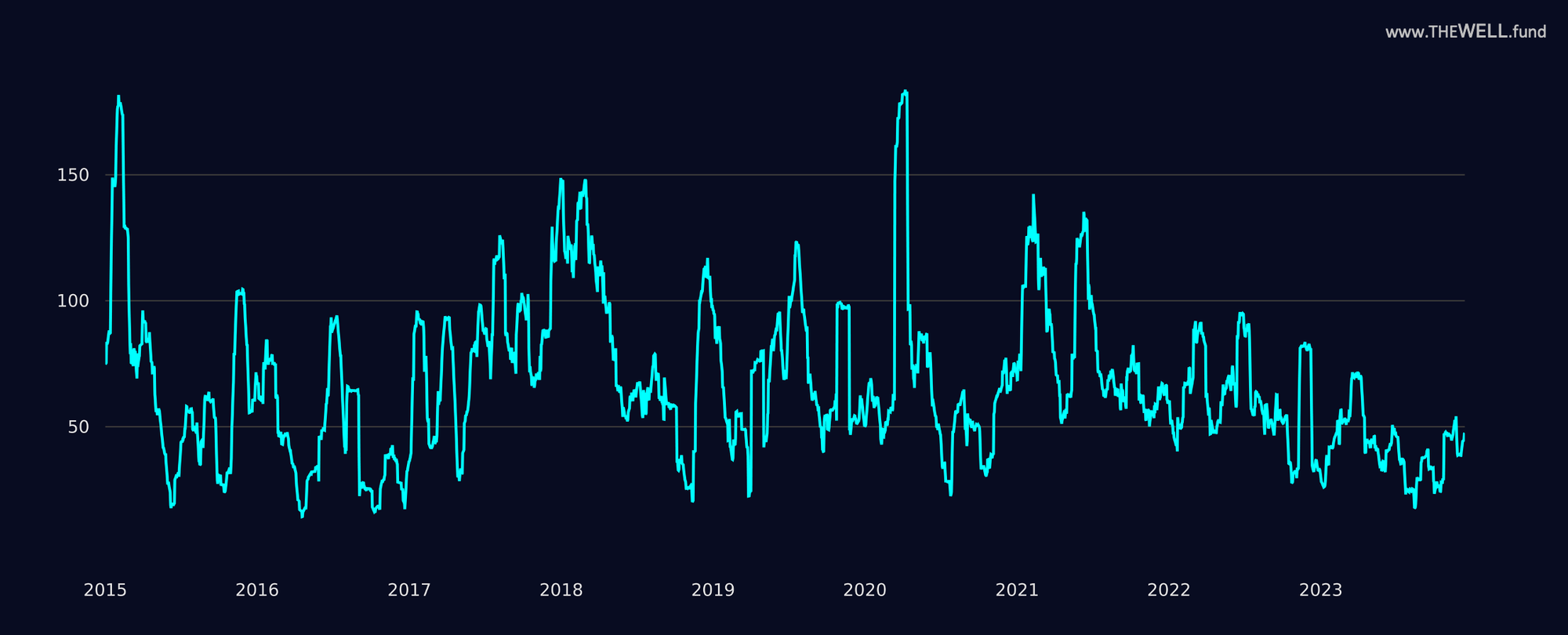
As volatility is one of the major factors contributing to the performance of momentum strategies, we believe that the most favourable environment is still ahead of us. Volatility barely picked up from recent historic lows and our models were able to capture large portions of the trends.
Investment portfolios that enjoyed outsized gains by being exposed to market beta this year may benefit from reduced risk by investing in a low volatility strategy that tends to profit from rising market volatility.
AI and its appetite for quant strategies
As the year draws to a close and AI has become the buzzword of the year, we, as a fund that employs machine learning and other AI tools, would like to share our perspective on AI in trading.
To get a clear start, it is worth noting that most advances in AI that get attention today come from the generative space. This implies the generation of novel data from an abstraction of existing data, thereby enabling models to be "creative" and comprehend unobserved context, which is truly remarkable. In many instances, one can say that those models learned reasoning.
Nonetheless, this is explicitly different from the task of forecasting or even nowcasting. Most tasks in quantitative models have little to do with generative tasks. Tasks in the realm of defining asset allocation exposures, mathematically defining a trend, mean reversion, etc. will most likely be significantly easier to do in any traditional way than a generative one.
Transformer models took over several tasks over the previous years that were already quite impressively executed by algorithms: translation, summarization, image and object recognition, data cleaning and generation, and many more. In our opinion, there are a few interesting tasks one can derive from the latest advancements.
- Data generation:
A big pain point in building models is having reliable assumptions about correlations. Many mathematical approaches were developed over the years (Ledoit-Wolf, Eigenvalue Cleaning, etc.), but a novel approach is to generate non-existing data to optimize on more robust assumptions on correlation behaviours.
- Pattern recognition:
Using generative models like GANs worked reasonably well during the last iteration of AI models and was the core of some “true AI” hedge funds. We can imagine even better results with some of the research results derived from other domains.
- News classification:
At every FED or ECB meeting, many attempts are made to translate their speeches into market directions. A recently published paper showed impressive results in classifying Hawkishness and Doveishness using models like GPT-3.5.
- Data cleaning:
Having experience with the usage of transformer models for cleaning and refilling missing data in another domain, an abstraction into financial data does not seem to be a far stretch and could be helpful for higher frequency forecasts heavily relying on data quality.
Besides direct deployment as alpha-generating models, we also see use cases in more operational tasks:
· Research
We found LLMs (large language models) very useful in helping us understand research, e.g., from other domains, and it is not easy to imagine Agents and Tools being ready to fulfil many more research tasks (e.g. utilizing AutoGPT or BabyAGI).
· Information retrieval
We have already utilized LLMs to use Q&A on long or sometimes hard to read documents (like contracts, etc.) and can see an enormous advantage, e.g., for bond, PE, or VC portfolio managers to understand and compare legal structures in an advantageous way.
There are a lot of other use cases we cannot even imagine right now, and we hope the above highlights the direction in which we are thinking.
As always, we can go into significantly more detail in a private conversation and welcome your thoughts and feedback.
This blog post is shared with the intention to provide educational content, general market commentary or company specific announcements. It does not constitute an offer to sell or a solicitation of an offer to buy securities managed by The Well GP.
Any offer or solicitation may only be made pursuant to a confidential Private Placement Memorandum, which will only be provided to qualified offerees and should be carefully reviewed by any such offerees for a comprehensive set of terms and provisions, including important disclosures of conflicts and risk factors associated with an investment in the fund.
Past performance is not necessarily indicative of or a guarantee of future results.
The Well GP makes no representation or warranty, express or implied, with respect to the accuracy, reasonableness or completeness of any of the information contained herein, including, but not limited to, information obtained from third parties. The information contained herein is not intended to provide, and should not be relied upon for accounting, legal, tax advice or investment recommendations.
This publication may contain forward-looking statements that are within the meaning of Section 27A of the Securities Act of 1933, as amended (the “Securities Act”), and Section 21E of the Securities Exchange Act of 1934, as amended (the “Exchange Act”). These forward looking statements are based on the management’s beliefs, as well as assumptions made by, and information currently available to, management. When used in this presentation, the words “believe,” “anticipate,” “estimate,” “expect,” “intend” or future or conditional verbs, such as “will,” “should,” “could,” or “may,” and variations of such words or similar expressions are intended to identify forward-looking statements. Although the management believes that the expectations reflected in these forward-looking statements are reasonable, we can give no assurance that these expectations will prove to be correct.